on JSTOR
on JSTOR
JSTOR, a nonprofit service from ITHAKA, invites you to explore the beta release of a new generative AI-powered research tool. We are excited about the collaborative possibilities ahead and aim to ethically, responsibly, and credibly use generative AI to empower people to deepen their research and unearth new avenues for discovery.
-
About JSTOR's AI research tool (beta) -
ITHAKA's approach to generative AI -
Frequently asked questions -
Legal notices
Do you have questions, comments, or concerns? We want to hear from you! Please email
Our generative AI-powered research tool is designed to help people work more efficiently and effectively. This beta feature will appear on the content page for journal articles, book chapters, and research reports, and as an alternative to JSTOR's standard keyword search. The tool helps you do the following:
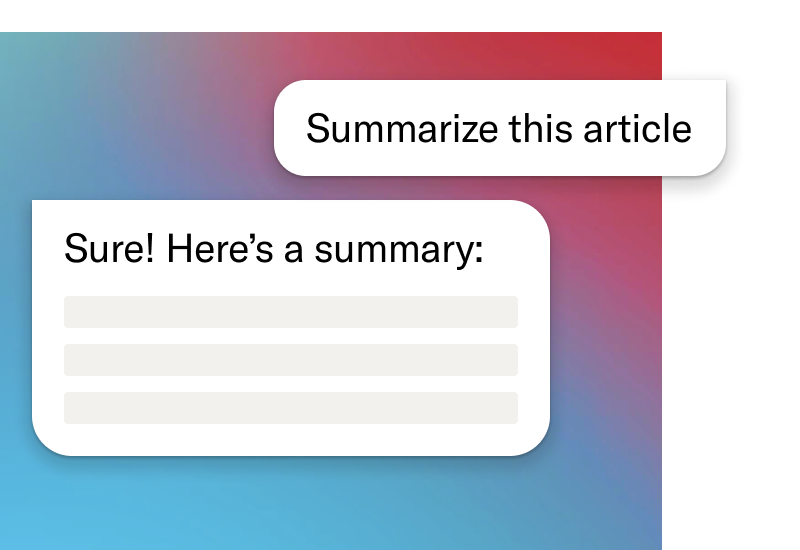
The tool generates a summary of what you're reading to help you quickly assess its relevance, and lets you know how it relates to your search terms
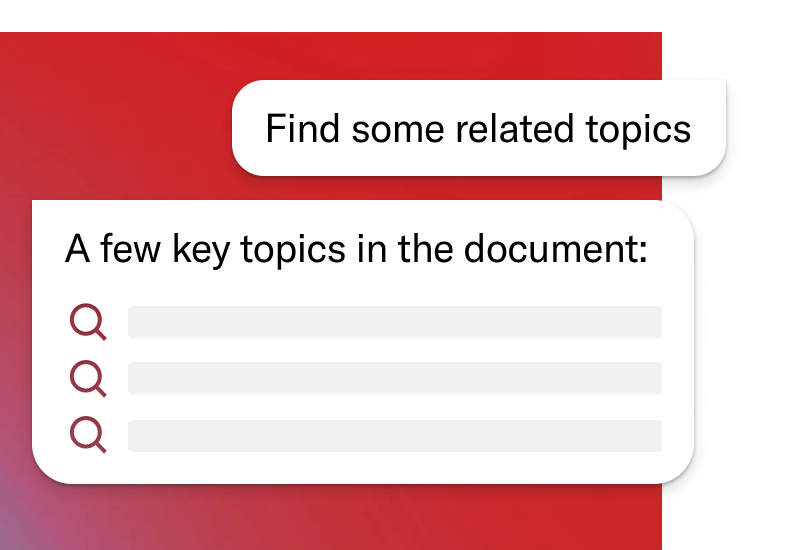
Discover related topics, enrich your reading with similar content from the JSTOR corpus, and try new ways of searching

Use natural language to ask questions and get quick answers about what you're reading or researching
ITHAKA offers a portfolio of nonprofit services, including JSTOR, Portico, and Constellate, aligned around a shared mission to improve access to knowledge for people around the world as affordably and sustainably as possible.
Technology plays a pivotal role in how ITHAKA achieves this aim. Through the research of
JSTOR and ITHAKA's founding president, Kevin Guthrie, shares his thoughts about our mission-driven approach to deploying new technologies to improve the learning and research experience for JSTOR users.
Ithaka S+R is collaborating with 20 colleges and universities on a multi-year research project to chart a productive path in the use of generative AI in higher education. We will be publishing three public reports related to the project's findings, as well as news along the way.
Just getting started with AI on JSTOR, or need a refresher? Visit our blog for an overview of what the tool can help you do.
What does JSTOR's generative AI research tool do?
- Generate a summary with key points and arguments from the text itself, helping users quickly determine if content is relevant to their research
- Suggest topics and show related content within the JSTOR corpus that is relevant to the text, enabling exploration of additional possible paths of inquiry
- Answer questions posed by users based only on the content of the document being viewed
- Search JSTOR in a new way with a semantic search-powered capability that works better for natural language queries than traditional keyword search
What content can the tool be used with?
What data sources is the tool drawing from to generate content?
Which large language models are JSTOR using for this generative AI-powered tool?
How do you plan to measure or ensure accuracy?
- Subject matter experts from a range of academic disciplines conduct in-depth, ongoing evaluations of the tool's output. These evaluations help us ensure that generated content is useful and accurate.
- Users provide in-tool feedback that we use to identify areas for improvement. All interactions with the beta tool offer users the opportunity to provide a thumbs up or thumbs down rating. For thumbs down ratings, the user can provide further detail to explain their response.
- We assess model performance for our specific use cases using industry-standard metrics for Machine Learning (ML) and Natural Language Processing (NLP). Additionally, we continuously integrate new evaluation metrics specifically developed for Large Language Model (LLM) use cases.
How will my information be kept, shared, and/or used?
JSTOR handles all personal information, including information provided to this tool, in accordance with our
Any data you provide to this tool, such as question prompts and other conversation data, will be stored in JSTOR's internal systems and used in de-identified form to maintain and improve the tool.
Your prompts and some/all of the text of the content being viewed is also sent to OpenAI to generate the response.
OpenAI does not use this to further train their models, nor do they retain the data for more than 30 days, in accordance with
Your data will be used in this way only if you opt in to the beta testing program.
Will there be fees associated with the generative AI tool?
How is content protected from unauthorized or malicious use?
JSTOR maintains physical, technical, and administrative safeguards to protect the content we hold.
We are a
Content is only processed internally and with the OpenAI API.
Please note the OpenAI API only temporarily stores data for the purpose of processing and does not use such submitted data to train their models or improve their service offering.
For more information on OpenAI's data security practices, please consult the
What is JSTOR's overall approach to generative AI?
Technology has always been an incredible accelerator for ITHAKA's mission to improve access to knowledge and education. As a trusted provider of scholarly materials, we have a responsibility to leverage our content, technology, and deep subject matter expertise to help our community of librarians, faculty, and students find paths to responsible, ethical, and productive uses of these tools.
- We honor our values first and foremost. JSTOR provides users with a credible, scholarly research and learning experience. Generative AI must enhance that credibility, not undermine it.
- We will listen closely and proceed cautiously. We recognize the concerns associated with generative AI and are pursuing this work mindful of the very real ethical, legal, and practical considerations at hand. Our first step is to deepen our collective understanding through research and by doing – and as always, in close collaboration with our community. We will use these tools safely and well.
- We empower people, we do not replace them. These tools should not be used to "do the work." They should be designed to help people, especially students, learn and do their work more efficiently and effectively.
- We will enable our systems to interact with users in ways that are comfortable. Traditionally, it has been the users' responsibility to adapt to restricted language and structures to provide computers with inputs; computers can now interact effectively with users in natural language, and we should take advantage of that.
- We will lead with care. We will deeply consider the aspirations and trepidations of the many communities we serve.
As we learn about and pursue this latest technology, we look forward to your engagement and insights to ensure we continue to deliver high-quality, trusted, impactful services that improve access to knowledge and education for people everywhere.
What does "limited beta" mean?
JSTOR's generative AI tool is referred to as a "beta" feature because it is still in rapid development and in need of user feedback to help us develop it into the best tool it can be. By "limited" we mean that access to this feature will be offered to a limited number of testers to ensure that our product and technology teams can best understand how users interact with these new features.
During the beta phase, we will work with engaged users to explore capabilities, foster innovation, refine functionality, and reveal limitations. As the tool evolves based on this work, its features may change. In this early stage of development, the ability of the tool will be limited, and its functionality less stable than other tools on JSTOR. In addition to product research and refinement, the following will occur:
- Quality assessment: Generating high-quality output with generative AI models can be challenging, as they may produce incorrect or undesirable results in certain scenarios. By limiting the user base initially, we can better manage output quality and ensure generated content aligns with our standards before exposing it to a broader audience.
- Feedback: The limited rollout enables us to gather valuable feedback from our community. This feedback helps identify and address any concerns, issues, or sensitivities before scaling up.
- Ethical and legal considerations: Limiting the user base at the start allows us to explore areas of concern, make policy decisions based on experience, evaluate potential risks, and implement necessary precautions.
- Scalability and infrastructure: Generative AI models require substantial computational resources to generate outputs. By initially limiting the number of users, we can ensure our infrastructure handles the demand while maintaining a stable user experience. Gradual expansion allows us to monitor and optimize systems before opening them up to a larger user base.
How do I volunteer to participate in beta testing?
Users who are
Note that access to our testing environment will be limited so we can create a controlled learning experience where our product and technology teams can best study user interaction with these new features. We are not able to guarantee access to the tool.
When will I get access to the beta tool?
We are not able to provide a timeframe for access to the tool. We will notify you if and when access to the pre-release features becomes available for you to explore.
While we aspire to offer more open and immediate access in the future, it's important that we limit user access in these early stages of development. This will allow us to:
- Manage performance and ensure a good experience
- Gain initial feedback from users
- Improve the experience in reaction to feedback
Will everyone get access to the tool eventually?
Please keep the following in mind as you explore generative AI on JSTOR.
By using JSTOR's generative AI tool you consent to JSTOR collecting any data that you choose to share with the tool.
JSTOR retains your conversation history in our logs and uses it in de-identified form, in accordance with our
Any data that is sent to OpenAI (which includes your prompt as well as some or all of the text of the content being viewed) is used only for generating the response.
They do not use this to further train their models, nor do they retain the data for more than 30 days, in accordance with
This AI tool is currently in a beta state, therefore its features are subject to change without notice as we develop it. Current user experience may vary and may not be reflective of the tool's future capabilities. Users should not rely on its outputs without conducting independent research. The tool should not be used to seek professional advice, including but not limited to legal, financial, and medical advice.
This evolving beta feature is built on JSTOR's digital archive, which includes millions of items, spanning centuries and representing a wide range of ideas and perspectives. Given the historical nature of the materials on JSTOR, content items will reflect the era in which they were produced and/or the perspectives of the content creators, including the language, ideas or other cultural standards of the time. Some content items may consist of or contain outdated language and ideas that are no longer in use and may be considered offensive, and the beta feature may repeat this when it summarizes or describes that content. Such responses reflect the views of the original content creators through the underlying content and not the views of JSTOR or its employees.
Any suggestions, ideas, or other information you would like to share regarding the tool may be used to improve, enhance, or develop its features. By submitting user feedback, you agree that such feedback becomes the sole property of JSTOR, and waive any rights, including intellectual property rights, related to the feedback.